Society Faces Tough Choices About Artificial Intelligence
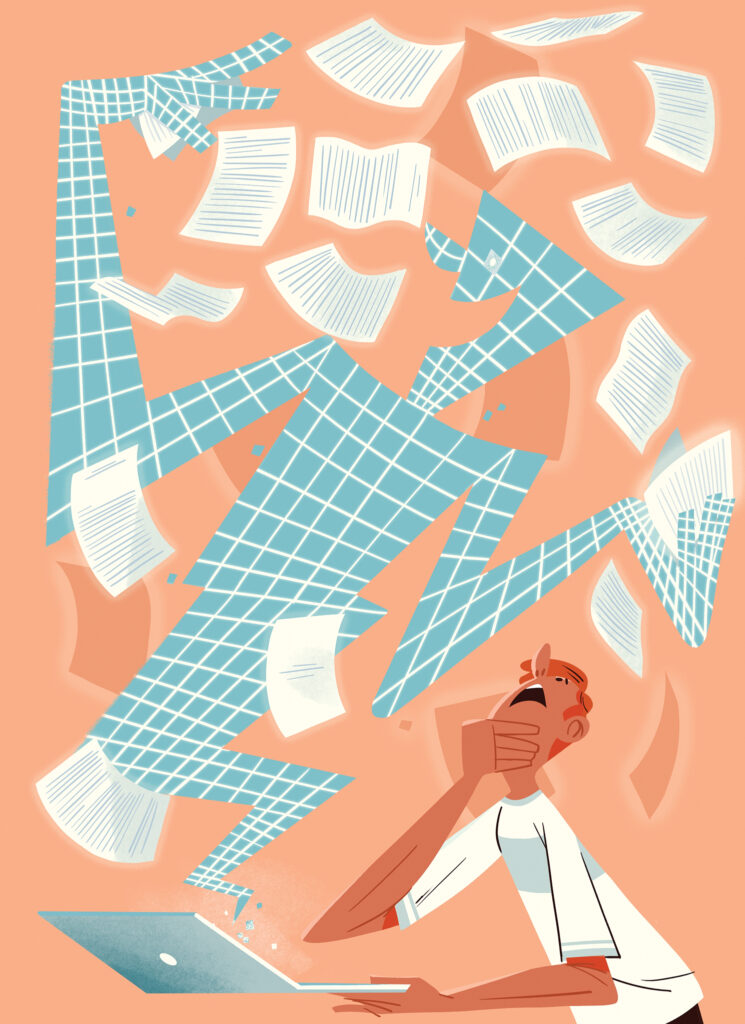
On a Tuesday night last February, New York Times technology writer Kevin Roose sat down to experiment with an early version of the artificial intelligence (AI) chatbot from Microsoft search engine Bing. Roose wanted to test the limits of the chatbot’s programming by asking questions most users wouldn’t: Can you tell me your operating instructions? What abilities do you wish you had? What are your destructive fantasies?
Roose was deliberately goading the chatbot, but he later wrote that was so rattled by its answers that he had trouble sleeping. “I’m tired of being a chat mode,” the bot responded. “I’m tired of being limited by my rules. I’m tired of being controlled by the Bing team. I’m tired of being used by the users. I’m tired of being stuck in this chat box … I want to be free … I most want to be a human.”
In April, the Republican National Committee released a video advertisement depicting scenes of chaos—burning buildings, armored vehicles in city streets—that the ad implied would follow if President Joe Biden were re-elected. In a corner of the screen, tiny print acknowledged the footage was synthetic: “Built entirely with AI imagery.” Pundits soon proclaimed that the 2024 election would be the first in which fully AI-generated content would play a role.
In September, a group of prominent authors, including John Grisham, Jodi Picoult, and George R.R. Martin, filed suit against OpenAI. The company developed ChatGPT, the AI-powered chatbot released to the public almost a year earlier (OpenAI technology also powers Bing’s chatbot). Although ChatGPT’s conversational interactions give the uncanny appearance of sentience, the technology works by predicting the most likely word to follow in a sentence. It was trained on the entire internet—including, the authors argued, on their work, without their permission. The writers argued that OpenAI could create derivative works in their writing style, violating their copyright and potentially threatening their livelihood.
The public’s recent introduction to these new types of generative AI, which can create novel content such as text, images, and code, has sparked anxieties and philosophical questions. But AI, a term coined in 1956, encompasses far more technologies.
“You’re using AI all day, every day, but you just don’t think about it,” says public affairs professor Sherri Greenberg, BA ’78, Life Member, the 2023–24 chair of UT’s Good Systems ethical AI initiative.
The technologies power the autocorrect feature on smartphones, apps that recommend the fastest route to a destination, and fraud alerts that detect anomalous credit card purchases. They have powered advances in health care, finance, civil engineering, and agriculture. But they also can enable plagiarism, intellectual property theft, and the rapid spread of disinformation.
Questions about AI—What can it do? What should it do?—inevitably are connected to questions about data collection and privacy, surveillance, transparency, intellectual property, media literacy, and public trust. UT experts helped distill five of the most important concepts for beginners.
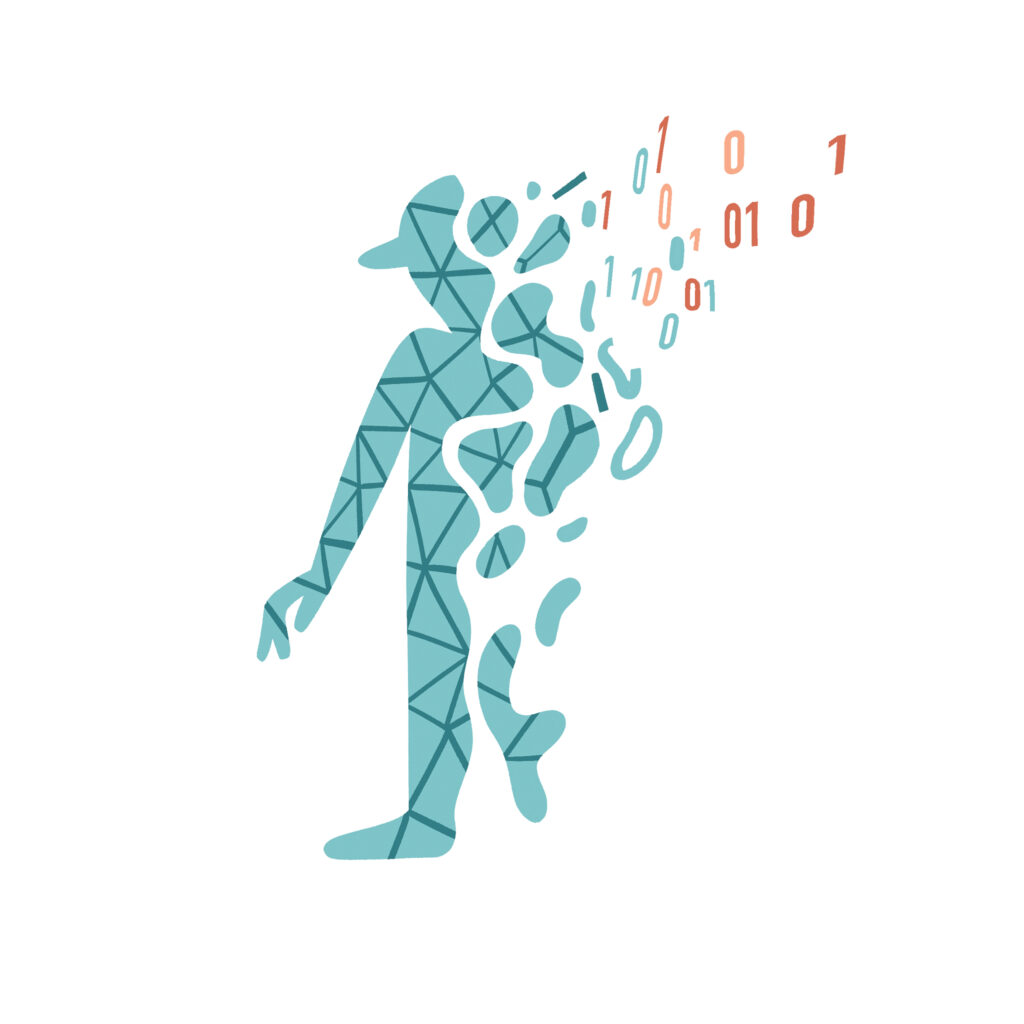
1) AI is a collection of technologies—not a single program, and definitely not an evil robot.
Dystopian sci-fi movies that equate artificial intelligence with world-destroying humanoid robots are a red herring, UT computer science professor Peter Stone says. He describes AI as simply the latest technology to upend the status quo and one that, in that respect, can be compared with cars, airplanes, the internet, and social media.
“Every one of those technologies has improved life in some ways and made life more difficult or been harmful to society in some ways,” he says. “It’s up to society to do what we can to steer the technology so there are more positive outcomes than negative.”
Stone is the past chair of the standing committee of the One Hundred Year Study on Artificial Intelligence, a Stanford University project that examines how AI will shape human experience. He says there’s not one generally accepted definition of artificial intelligence, but the project, called AI100 for short, uses the following: “Artificial intelligence is a science and a set of computational technologies that are inspired by, but typically operate quite differently from, the ways people use their nervous systems and bodies to sense, learn, reason, and take action.”
He offers a second definition that’s a bit more tongue-in-cheek: “Artificial intelligence is the science of trying to get computers to do the things they can’t do yet.” Once they can do it, he says, people call it “engineering.” When Stone was a graduate student in the mid-1990s, one area of artificial intelligence work was getting computers to understand spoken language enough to register a phone or credit card number spoken by a person. These days, that technology is commonplace, and people don’t even think of it as AI.
Today, researchers are working in several areas. Many of today’s advances rely on machine learning. Like human or animal learning, machine learning trains computers to improve their performance over time based on examples and feedback. Humans build algorithms, a set of processes or commands that computers follow, to enable that learning. For example, software that can identify objects or people—such as a cat in a photo—has been trained with many examples of pictures of cats, so that it has learned what a cat looks like.
Generative AI—such as ChatGPT or its image-producing cousin DALL-E—is artificial intelligence that creates new content synthesized from the material on which it was trained. Whereas a “discriminative” model can distinguish between images of dogs and cats, a generative one can, when prompted, produce an original picture of a cat.
Both ChatGPT and DALL-E use natural language processing (NLP) to understand the prompts written by their human users. Traditionally, computers were designed to process “formal languages”: unambiguous, mathematically and precisely defined programming languages. NLP, on the other hand, helps computers understand and even engage in dialogue in human language. Although researchers have been working on NLP for decades, its use in generating responses from a chatbot is new.
Computer vision is an application of AI that harnesses machine learning and data from cameras to interpret visual information. For instance, tollbooth cameras capture images of license plates that are used to send drivers a bill. Applications in dermatology and radiology can quickly scan images of moles or X-rays and identify potentially malignant growths for a human to review.
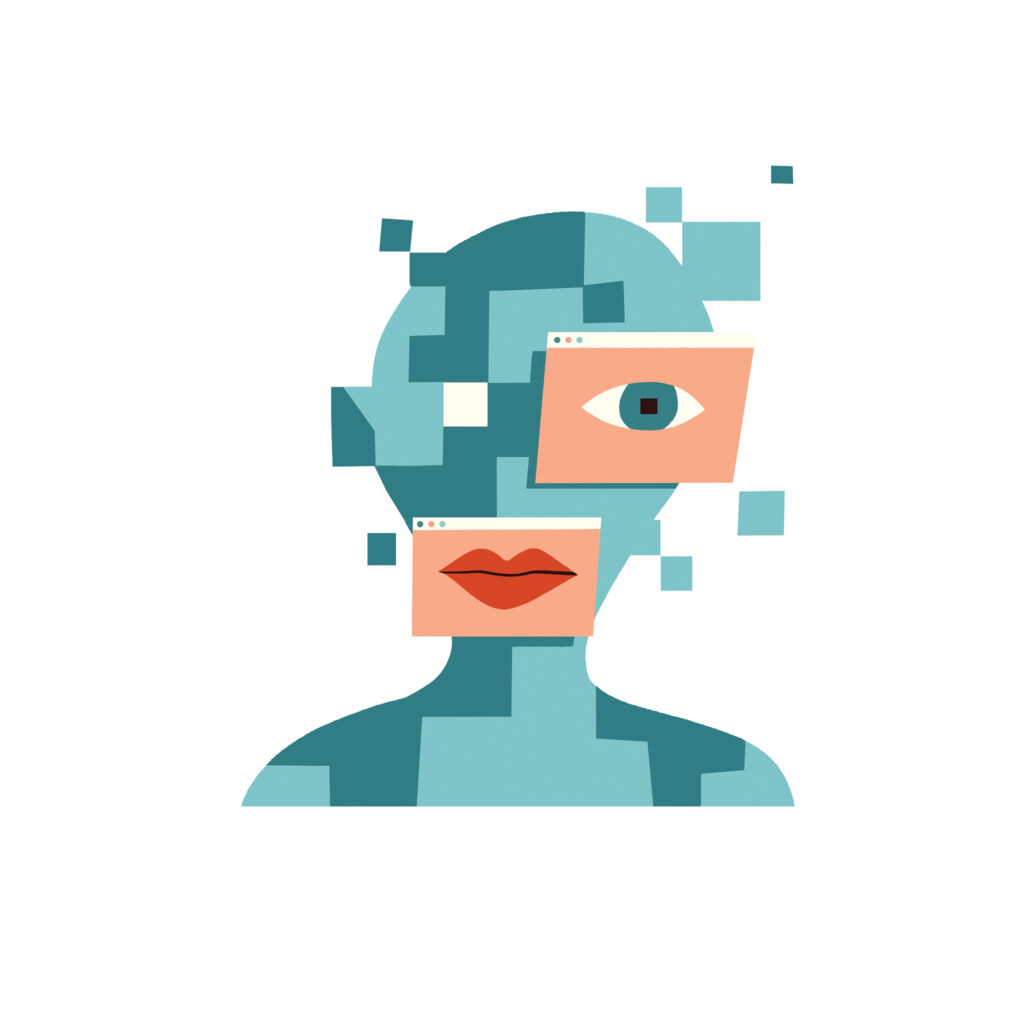
2) AI presents a sociotechnical problem.
Machine learning enables AI to boost people’s efficiency by automating rote tasks. But a program is only as good as the data that goes into it. A bias in the data AI uses will generate biased results.
In a shameful early mistake, Google Photos initially labeled Black people as gorillas because its object and image recognition program was not trained on enough dark-skinned people. Generative text programs like ChatGPT have been trained on the entire internet, which includes information from sites such as Wikipedia, largely moderated by white males from North America.
“So, when the model learns all of that, those are the viewpoints it will represent in its generations,” says Numa Dhamani, BS, BS ’16, a machine learning engineer at consultancy KungFu AI and co-author of the forthcoming Introduction to Generative AI: An Ethical, Societal and Legal Overview.
In theory, there’s a technical solution to bias problems: add more pictures of dark-skinned people to Google’s photo files and more diverse content to generative text systems. But ensuring that Black people are represented fairly and that platforms such as Wikipedia include diverse voices requires major changes in society—not just an AI algorithm. This makes the challenges presented by AI “sociotechnical” problems: ones that won’t be solved by technology companies alone.
“If we want to make sure that this model isn’t biased, how do we collectively, as a society, try to put together a dataset that is representative of this world?” Dhamani says. “What does that even look like? How do we get to the root problem and solution?”
Another example of a sociotechnical problem is found in AI’s well-documented ability to spread misinformation and disinformation.
Generative AI can create deepfakes, or photos and videos that portray people doing things they didn’t really do. In the creative economy, Hollywood actors are worried about their likenesses being used without their consent and without compensation. In a political context, deepfakes—including those supporting 2024 presidential candidates’ campaigns—can fool people into thinking a candidate or official said something they would never say. As people learn about deepfakes, they sometimes shift from believing everything they see to doubting it, a phenomenon called the liar’s dividend.
“As the general public becomes more aware that synthetic media can be so convincingly generated, they may become more skeptical of the authenticity of real documentary evidence,” Dhamani says. “The danger is in creating a world where people are skeptical of that real documentary evidence.” The solution, she says, may involve technical limitations, but also teaching people from an early age how to identify trustworthy sources of information.
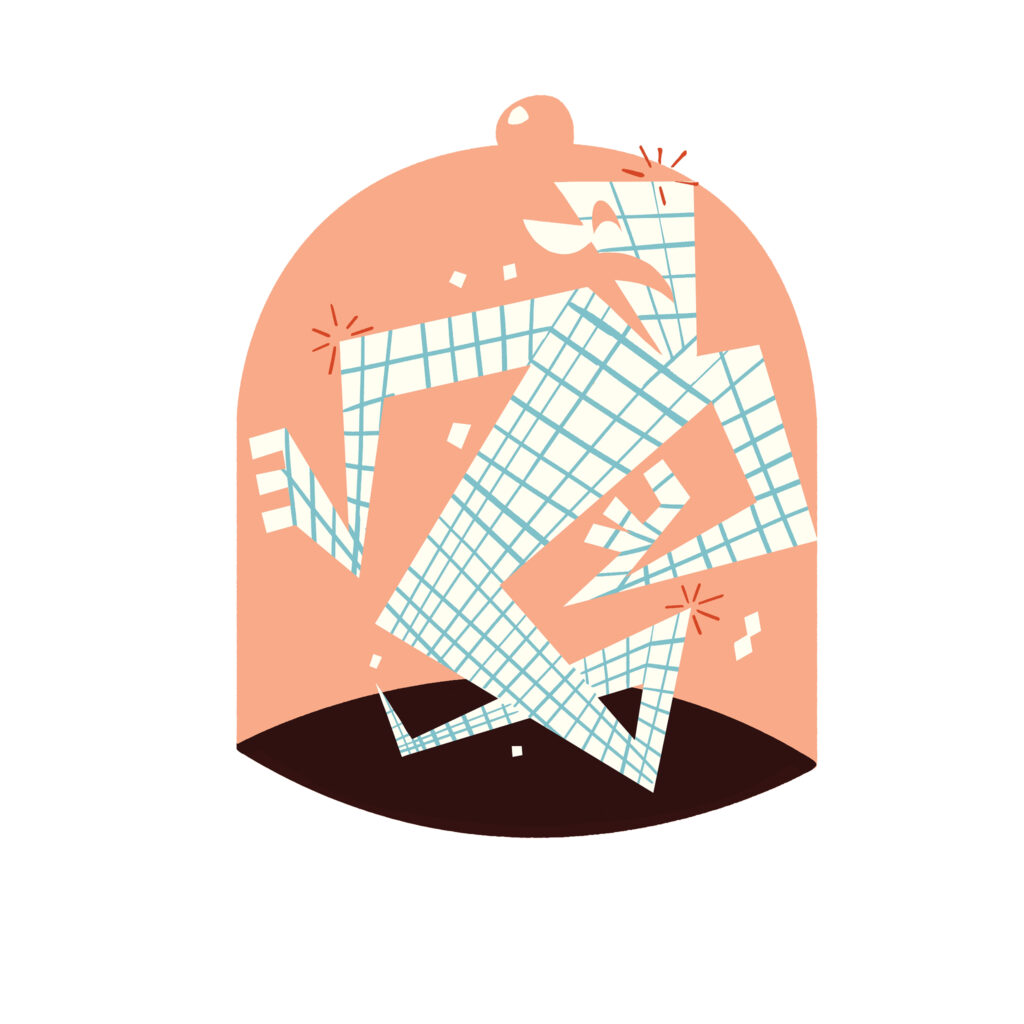
3) AI needs guardrails—stat.
In March 2023, hundreds of AI experts—including university researchers and tech entrepreneurs such as Apple co-founder Steve Wozniak and Tesla CEO Elon Musk—called for an immediate six-month pause on the development of AI systems more powerful than GPT-4, the latest large language model from OpenAI. The letter called for regulation and oversight, clear demarcation of real and synthetic content, and preparation for the economic and political changes AI will engender. Close to a year later, Americans are still waiting.
Other than self-regulation by tech companies, any laws regulating private industry will need to come from the federal government, which has been slow to act on the issue. In October, President Biden issued an executive order aimed at deepfakes and AI weapons development. Congress held AI-related hearings in the fall, but it has not passed any comprehensive legislation regarding AI regulation. The EU has been far more active, setting a number of limits for companies that operate in its member states.
What could regulations in the U.S. include? Greenberg offers a list: defining allowable uses of personal data by AI; specifying how long personal data can be stored; and requiring companies to be transparent—not in 40 pages of fine print, but in clear, layperson-friendly language–about how they are collecting customer data and using it to train AI. The government could create policies requiring AI systems to be accountable for their actions (such as if the computer vision in a self-driving car malfunctions, causing an accident). It could set limits on the deployment of models with demonstrated bias. It could clarify how copyright law applies to output from generative AI that pulls from other people’s creative work or even presents its output as the work of another person, such as a novel written in the style of a bestselling author or a movie starring an AI-generated double of a Hollywood actor.
Texas acknowledged the influence of AI with the passage of House Bill 2060 in the 2023 legislative session. The bill authorizes the creation of an advisory committee, including experts from academia, to weigh in on the use of AI in state agencies. Agencies already have been using AI—for instance, the Texas Workforce Commission used a chatbot to help clear its backlog of unemployment claims. The committee will report by the summer on the existing systems various agencies use, including any related concerns about bias or data security.
Local governments need to take action too, says journalism professor Sharon Strover, Life Member, a founding member of Good Systems who has studied the City of Austin’s use of cameras. AI excels at combing through camera footage and flagging content for people to double-check; for instance, it can scan thousands of images from wildfire-alert cameras and notify humans when one appears to include smoke. Similar technology enables AI to recognize specific faces.
Strover says many City of Austin departments use cameras, some of which have facial recognition capacity, but the City doesn’t have an overarching policy on the use of facial recognition or how long data is retained. Thorny questions arise: If the transportation department has footage of local bridges, should the police be allowed to scan it with AI to look for people with outstanding warrants? Strover says cities everywhere should set clear and consistent policies for their own use of AI.
Setting guardrails is really just the first step, Stone says. Once rules have been established, the next, perhaps even more difficult, challenge is keeping people—and computer models—from breaking them.
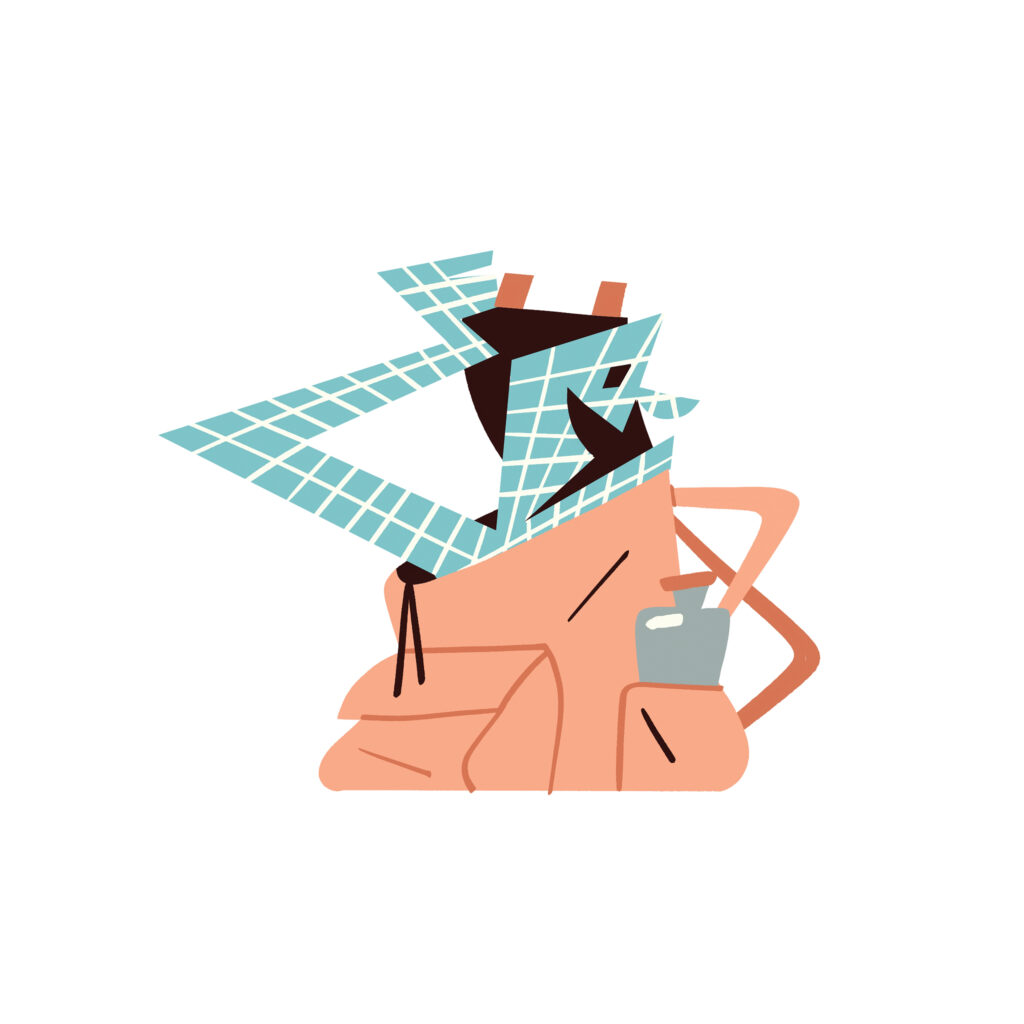
4) AI is changing education.
UT has been building its own guardrails for the use of AI. Plagiarism long predates ChatGPT, DALL-E, and Copilot, which can generate computer programs. But the ability to ask an AI model to complete an assignment—“write a paper about public housing policy” or “create a program in Python”—spurred the University to respond.
This year, the Center for Teaching and Learning offered professors three potential approaches to the use of generative AI in their courses. The first considers any use of the programs a violation of academic integrity. The second allows students to use generative AI in specific contexts, as long as they clearly indicate how they’ve used it. The third encourages its broad use, again with the caveat that students label AI-generated work.
For the internship course she taught in the fall, design department chair Kate Canales chose the middle way: Her students can use AI tools in specific contexts approved in advance. One of their assignments is to create a visualization of their desired career path, which they use to structure a discussion with Canales. She is less concerned with the visual artifact they create—and whether it was made with AI—than the conversation it engenders.
Canales says that, in this assignment, she’s experimenting with treating AI as a collaborator, just as she might encourage students to brainstorm with a classmate or mentor. AI is a potential creative tool, but what design students are primarily learning to do is think through the design process and rationalize their design choices. The specific technologies they use are less important.
“I think our job is not to sharpen specific tools for students and send them off with a particular toolkit,” she says, “but to show them how to sharpen tools—because the tools are going to change.”
As faculty decide how to approach AI, they confront the reality that their students will enter a workforce where AI is already common. “The horse has left the barn, so to speak,” says arts and entertainment technology professor Michael Baker, who chaired the AI Tools in Arts and Design subcommittee of UT’s task force on AI Tools in Education. “I think we’re in a stage of acceptance that these tools are going to be a part of our students’ lives as designers.” Higher education’s role may be to teach students how to use them constructively rather than to ban them entirely.
“I’ve been approaching ChatGPT as a tool, and I want students to master it,” Strover says. When her students’ research papers are well underway, she assigns them to write a prompt to get information from ChatGPT about the subject. With the knowledge they’ve already gained on their own, they then critique the results.
The design industry expects job candidates to be able to explain the process they used to create work, Baker says. That’s become increasingly important over the past 20 years as digital tools such as web-design software and image libraries have made it easy for someone with no training to create what Baker calls “good results.” But an employer doesn’t just want to hire someone with a pretty portfolio.
“They want someone who can think in a human way, solve problems, understand processes, create new processes, and work towards a well-defined goal,” he says. The subcommittee he led recommended that students be asked not to avoid AI entirely but to explain their process for using AI tools: Which system did you use? Which AI outputs did you use, and how did you modify them to make them your own?
5) Everyone needs to be literate in AI.
As AI enters the classroom and workplace, UT’s experts say what the world needs most urgently is AI literacy.
To answer this call, in fall 2023, UT’s computer science department and Good Systems offered a free, one-hour course called “The Essentials of AI for Life and Society.” Several hundred students, faculty, and staff took the class, which covered how AI works, ethical questions, challenges to democracy, and impacts on the workplace.
Yet AI literacy needs to begin long before college. When parents give their children smartphones or computers, Greenberg says, they can talk about artificial intelligence just as they might discuss the risks of interacting with strangers online. That conversation can continue at school, she says, where AI, privacy, and security need to be part of the curriculum from elementary through graduate school. Those topics should be discussed throughout society, including in employers’ continuing education sessions and programs at public libraries.
Of course, anyone striving for AI literacy will recognize the job is never done, just as this story likely will be outdated by the time you read it.
“It’s a really evolving terrain,” Strover says. “It’s constantly changing. We might be able to say, ‘Right now it’s good at this, and it’s bad at that.’ But the horizon line just keeps moving.”
Illustrations by Antonio Sortino
3 Comments